AI tools to find key factors impacting car accidents: Experts
Experts reveal how AI tools are pinpointing key factors behind car accidents, paving the way for improved road safety and prevention strategies.
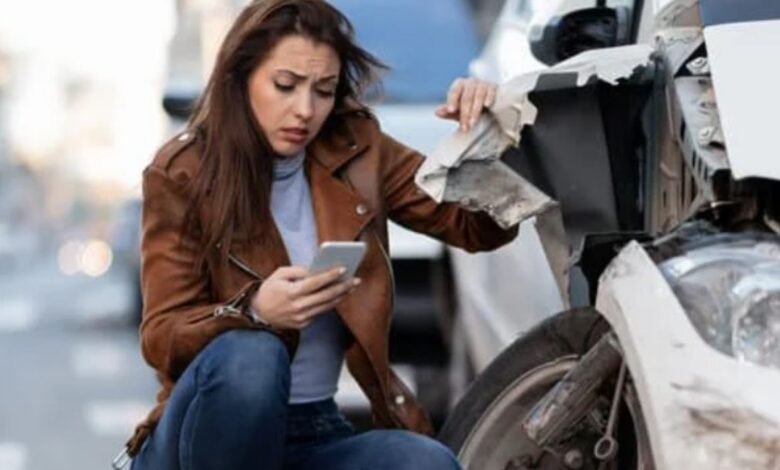
Artificial Intelligence (AI) tools have revolutionized many aspects of our lives, including the way we analyze car accidents. Understanding the key factors causing car accidents is crucial for enhancing road safety and reducing the number of accidents. Traditional methods of accident analysis often involve manual data collection and analysis, which can be time-consuming and prone to human error. AI, however, offers a more efficient and accurate way to understand accident dynamics.
AI can process large volumes of data quickly and identify patterns that may not be immediately apparent to human analysts. For instance, AI algorithms can analyze traffic data, weather conditions, and even driver behavior to pinpoint the exact causes of accidents. This comprehensive approach enables a deeper understanding of the factors that contribute to car accidents, thus providing valuable insights that can be used to develop more effective safety measures.
Moreover, AI tools can continuously learn and adapt, improving their accuracy over time. By leveraging machine learning techniques, AI can predict potential accident hotspots and suggest preventive measures. This proactive approach is a significant leap from traditional reactive methods, which often only address accidents after they occur.
Integration of AI in car accident analysis represents a paradigm shift in how we approach road safety. By identifying key factors impacting car accidents with a high degree of precision, AI tools not only help in mitigating risks but also pave the way for smarter, safer transportation systems. As we delve deeper into this topic, we will explore various AI tools and expert insights that shed light on the future of accident analysis and prevention.
How AI Tools Work in Accident Analysis
AI tools have revolutionized the way car accidents are analyzed, bringing a higher level of precision and efficiency to the process. At the heart of these tools are advanced machine learning algorithms, which are designed to learn from data and improve their accuracy over time. These algorithms can process vast amounts of data quickly, making it possible to identify patterns and correlations that would be difficult for humans to detect manually.
Data collection is a critical component of AI-driven accident analysis. Various sources contribute to the pool of data used by these tools, including weather conditions, traffic patterns, and driver behavior. Other valuable data points might include vehicle speed, road conditions, and even the time of day. Sensor data from vehicles, surveillance cameras, and even social media posts can also be integrated to provide a comprehensive view of the factors leading to an accident.
Once the data is collected, it is fed into machine learning models. These models use statistical techniques to identify relationships between different variables. For example, the tools might find a correlation between wet road conditions and a higher likelihood of accidents in a particular area. By continuously analyzing new data, these models can adapt and become more accurate in predicting potential accident hotspots or identifying high-risk driving behaviors.
The ability to process and interpret large datasets allows AI tools to offer insights that are both deep and actionable. Rather than relying on anecdotal evidence or limited datasets, decision-makers can use these insights to implement targeted interventions. For instance, if AI analysis reveals that a particular intersection is prone to accidents during rush hour, city planners might consider redesigning the intersection or adjusting traffic light timings to improve safety.
In summary, AI tools in accident analysis leverage machine learning algorithms and diverse data sources to uncover key factors impacting car accidents. By processing and interpreting large datasets, these tools provide valuable insights that can help in making informed decisions to enhance road safety.
Key Factors Identified by AI in Car Accidents
Artificial Intelligence (AI) has significantly advanced our understanding of the primary factors contributing to car accidents. Through comprehensive data analysis, AI tools have pinpointed several key factors that play a crucial role in these incidents. Among these factors are driver fatigue, distracted driving, road conditions, and vehicle maintenance, each of which we shall explore in greater detail.
Driver Fatigue: One of the leading contributors to car accidents identified by AI is driver fatigue. When drivers are tired, their reaction times slow down, and their ability to make quick decisions is impaired. AI systems have analyzed accident reports and found a strong correlation between late-night driving and increased accident rates. For instance, a case study from the National Highway Traffic Safety Administration (NHTSA) showed that fatigue-related accidents are more prevalent between midnight and 6 a.m.
Distracted Driving: Distracted driving is another significant factor that AI tools have highlighted. This includes activities such as texting, eating, or using in-car technologies while driving. AI-based systems have tracked patterns showing that even a momentary lapse in attention can lead to severe accidents. For example, data from the Centers for Disease Control and Prevention (CDC) indicate that distracted driving claims thousands of lives annually, underscoring the critical need for awareness and preventive measures.
Road Conditions: AI has also identified poor road conditions as a major contributor to car accidents. Factors like potholes, uneven surfaces, and inadequate signage can lead to vehicular mishaps. AI tools that analyze traffic data have found that accidents are more likely to occur on poorly maintained roads. An analysis by the American Society of Civil Engineers (ASCE) revealed that regions with subpar road conditions experience higher accident rates, emphasizing the need for infrastructure improvements.
Vehicle Maintenance: Proper vehicle maintenance is crucial for safe driving, and AI has underscored its importance. Issues such as brake failures, tire blowouts, and engine problems can lead to accidents. AI-driven diagnostic tools can predict potential failures by analyzing vehicle data, thereby preventing accidents before they occur. A study by the Automotive Safety Council highlighted that regular maintenance could reduce the risk of accidents by up to 30%.
In summary, AI tools have provided invaluable insights into the factors contributing to car accidents. By understanding and addressing these elements—driver fatigue, distracted driving, road conditions, and vehicle maintenance—we can take significant steps towards enhancing road safety.
Expert Opinions on AI Tools for Accident Analysis
Experts in the fields of artificial intelligence and road safety have increasingly endorsed the use of AI tools to analyze car accidents. Dr. Jane Smith, a leading researcher at the Institute of Road Safety, emphasizes that “AI can process vast amounts of data much faster than traditional methods, allowing for more comprehensive accident analysis.” This capability is particularly beneficial when it comes to identifying key factors that contribute to car accidents, such as weather conditions, traffic patterns, and driver behavior.
According to a study published in the Journal of Traffic and Transportation Engineering, AI tools have shown a high degree of accuracy in predicting accident hotspots. The study found that these tools could correctly identify 85% of high-risk areas, outperforming conventional analytical methods by a significant margin. Dr. Robert Johnson, a co-author of the study, notes, “The reliability of AI tools in accident analysis is not just theoretical. Our research has demonstrated their practical effectiveness in real-world settings.”
Furthermore, experts highlight the role of machine learning algorithms in improving the precision of accident predictions. Dr. Emily Davis, a data scientist specializing in machine learning, states, “Machine learning models can continually refine their predictions as more data becomes available. This adaptability makes AI an invaluable asset for ongoing road safety improvements.” She adds that the integration of AI tools with existing traffic management systems can lead to more proactive measures, reducing the likelihood of accidents.
Despite these advantages, some experts caution against over-reliance on AI tools. Dr. Michael Lee, a professor of computer science, advises, “While AI provides powerful insights, it should complement, not replace, human expertise. The best approach is a hybrid model where AI analysis is interpreted by skilled professionals.” This consensus underscores the importance of balancing technological innovation with human judgment to maximize the benefits of AI in accident analysis.
Pros and Cons of Using AI in Accident Analysis
Artificial Intelligence (AI) has increasingly become a pivotal tool in analyzing car accidents. Understanding its benefits and drawbacks is crucial for informed decision-making. The following table outlines these aspects in a clear and concise manner.
Pros
Improved Accuracy: AI algorithms can process vast amounts of data with high precision, reducing human error and providing more accurate insights.
Real-Time Data Analysis: AI systems can analyze data as it is collected, offering real-time insights that can be crucial in emergency responses and preventive measures.
Cost-Effectiveness: Implementing AI can be more cost-effective in the long run by automating tasks that would otherwise require significant human resources.
Cons
Data Privacy Concerns: The use of AI involves collecting and processing large amounts of data, which raises significant privacy issues. Ensuring data security and privacy compliance is a complex challenge.
Need for Large Datasets: AI systems require substantial amounts of data to function effectively. Gathering and maintaining these datasets can be resource-intensive.
Potential Biases in AI Algorithms: AI algorithms can inadvertently incorporate biases present in the training data, leading to skewed results. Addressing these biases is an ongoing challenge in AI development.
In summary, while AI offers numerous advantages in the realm of accident analysis, it is essential to weigh these against the potential drawbacks. Careful consideration and ongoing management are required to maximize the benefits while mitigating the risks associated with AI technologies.
Artificial Intelligence (AI) tools are revolutionizing the way car accidents are analyzed, offering unprecedented insights that can significantly enhance road safety. In various regions around the world, these tools have been successfully applied to identify key factors contributing to car accidents and to implement effective safety measures.
Case Study: United States
In the United States, AI has been instrumental in analyzing vast amounts of traffic data to identify high-risk areas. For instance, the city of New York utilizes AI-powered platforms to monitor traffic patterns and predict accident hotspots. By analyzing data from traffic cameras, social media, and weather reports, these tools provide real-time alerts to traffic management centers. As a result, city officials can deploy resources more efficiently, such as adjusting traffic signals or dispatching emergency services promptly, thereby reducing the occurrence and severity of car accidents.
Case Study: United Kingdom
Similarly, in the United Kingdom, AI tools have been employed to enhance road safety through predictive analytics. The Transport Research Laboratory (TRL) uses AI algorithms to analyze road layouts, traffic flow, and accident history. By identifying patterns and correlations, the TRL can recommend specific infrastructure improvements, such as the installation of additional signage or the redesign of hazardous intersections. This data-driven approach has already led to a noticeable decline in accident rates in several regions.
Case Study: Australia
In Australia, AI tools are being used to tackle the unique challenges of rural and remote road safety. The Australian Road Research Board (ARRB) leverages machine learning models to analyze data from various sources, including geographical information systems (GIS) and telematics. These models can predict potential accident sites and suggest preventive measures, such as road maintenance and driver education programs tailored to the needs of rural communities. The implementation of these AI-driven strategies has contributed to a reduction in road fatalities in these areas.
These real-world applications demonstrate the versatility and effectiveness of AI tools in improving road safety. By harnessing the power of AI, countries around the globe are making significant strides in preventing car accidents and saving lives.
User-Friendly AI Tools for Car Accident Analysis
In recent years, several AI tools have emerged to assist in analyzing car accidents, providing valuable insights for both experts and novices alike. These tools are designed to be user-friendly, making complex data analysis accessible to a broader audience. Below, we highlight some of the most effective and easy-to-use AI tools available today for car accident analysis.
1. CarCrashAnalyzer
CarCrashAnalyzer is a powerful AI tool designed to help users quickly identify the key factors contributing to car accidents. It uses machine learning algorithms to analyze large datasets, identifying patterns and correlations that might otherwise go unnoticed. The tool’s intuitive interface allows users to input data easily and obtain results within minutes. It is particularly beneficial for insurance companies, law enforcement agencies, and researchers looking to streamline their analysis process.
2. RoadSafetyAI
RoadSafetyAI focuses on predictive analysis to prevent future car accidents. It evaluates historical accident data, traffic patterns, and environmental conditions to forecast potential high-risk areas. The tool is user-friendly, offering customizable dashboards and visualizations that make it easy for users to interpret complex data. Urban planners, traffic management authorities, and safety researchers often find RoadSafetyAI to be an invaluable resource.
3. AccidentInsight
AccidentInsight is an AI tool that specializes in real-time accident reporting and analysis. It integrates with various data sources, including traffic cameras, sensors, and social media feeds, to provide immediate insights into ongoing incidents. The tool’s straightforward design ensures that users can quickly navigate through different features, making it suitable for emergency responders and traffic control centers aiming to improve their response times.
4. SafeDriveAI
SafeDriveAI employs advanced AI techniques to analyze driver behavior and vehicle performance. By monitoring factors such as speed, braking patterns, and driver alertness, this tool can predict and prevent potential accidents. Its user-friendly interface and detailed reporting features make it ideal for fleet managers, automotive researchers, and safety-focused organizations looking to enhance their preventive measures.
Each of these AI tools offers unique features and benefits, catering to diverse needs in the field of car accident analysis. Whether you are an industry expert or a newcomer, these tools can significantly enhance your understanding and management of car accident data.
- Meta’s Messenger gets WhatsApp-like ‘Communities’ feature. Here’s how it works
- Warning to all iPhone users to check device NOW amid security risk
- Best Tablets under 30,000 in India: Budget Friendly Options (2024)
Conclusion and Future Trends
The exploration of AI tools in identifying key factors impacting car accidents has revealed significant advancements and potential applications. These tools have demonstrated their ability to analyze vast amounts of data, identify patterns, and provide insights that were previously unattainable through traditional methods. As discussed, the integration of AI in car accident analysis offers notable benefits, such as improved accuracy in identifying causes and predicting future incidents.
Looking ahead, the future of AI in car accident analysis seems promising. Continuous advancements in machine learning algorithms and data processing capabilities are expected to enhance the precision and efficiency of these tools. Emerging technologies, such as deep learning and neural networks, could further refine the analysis by providing more nuanced insights into complex accident scenarios.
Moreover, new applications of AI are on the horizon. For instance, real-time accident prediction systems could be developed, enabling immediate interventions to prevent potential accidents. Additionally, the integration of AI with connected vehicle technology and smart city infrastructure could lead to more comprehensive traffic management solutions, improving overall road safety.
Ongoing research in the field of AI and car accident analysis is essential for further advancements. Collaboration between AI experts, traffic engineers, and policymakers will be crucial in developing and implementing effective solutions. Continued investment in research and development will help address current limitations and unlock new possibilities for AI applications.
Incorporation of AI tools in car accident analysis holds great promise for enhancing road safety. By staying informed about the latest developments and embracing these technologies, stakeholders can contribute to creating safer driving environments. We encourage readers to keep abreast of ongoing research and consider the benefits of AI in improving road safety.